HeartLens
HeartLens has the potential to detect calcium deposits in the heart earlier, leading to timelier interventions and less invasive and aggressive patient treatment options.
Coronary artery calcification (CAC) scoring is a critical process in assessing cardiovascular disease severity. This process requires radiologists to manually review CT scans to identify small calcium deposits—subtle markers that can be easily overlooked. This is essential for early diagnosis and intervention, but it takes time and can be prone to human error.
Enter HeartLens, an AI-based tool designed to assist radiologists in reviewing CT scans, detecting CAC, and supporting cardiologists in making informed diagnostic and treatment decisions. By enabling earlier identification of coronary calcification, HeartLens can facilitate timelier, less invasive interventions, ultimately improving patient outcomes.
Developed as part of the University of Kentucky’s EXCEL Research Initiatives, HeartLens represents a partnership between clinical and research teams across the UK HealthCare Divisions of Radiology and Cardiology, the Department of Computer Science, and CAAI. This interdisciplinary collaboration ensures the model is clinically grounded and technically robust.
HeartLens is a task-specific adaptation of foundational computer vision models. At the core of our approach is DINO, a self-supervised Transformer-based vision model, with a U-Net architecture, a standard in medical image segmentation. We began by training DINO on publicly available CT scan datasets from Stanford. One befit of the DINO model is that is can learn visual patterns without the need for annotated, manually labeled data. The model was further fine-tuned using UK’s own imaging data to improve its ability to detect and segment calcified regions in the heart. Data collection, cleaning, training and testing were conducted over a 6 month period.
HeartLens locates, highlights, and labels CAC features in CT scans, giving clinicians clearer visual cues and improving their ability to assess risk. These extracted features can also support downstream tasks such as classification, segmentation, and future integration with large language models (LLMs) for further enhanced diagnostic accuracy and utility.
HeartLens is still in development. Next steps include clinical evaluation, where our partners will assess the impact of AI-assisted CAC detection on patient care and decision-making. As development continues, the goal is to integrate AI seamlessly into cardiovascular workflows supporting faster, more accurate diagnosis and expanding access to early intervention.
This effort is in progress and funded by the EXCEL Research Initiative at the University of Kentucky.
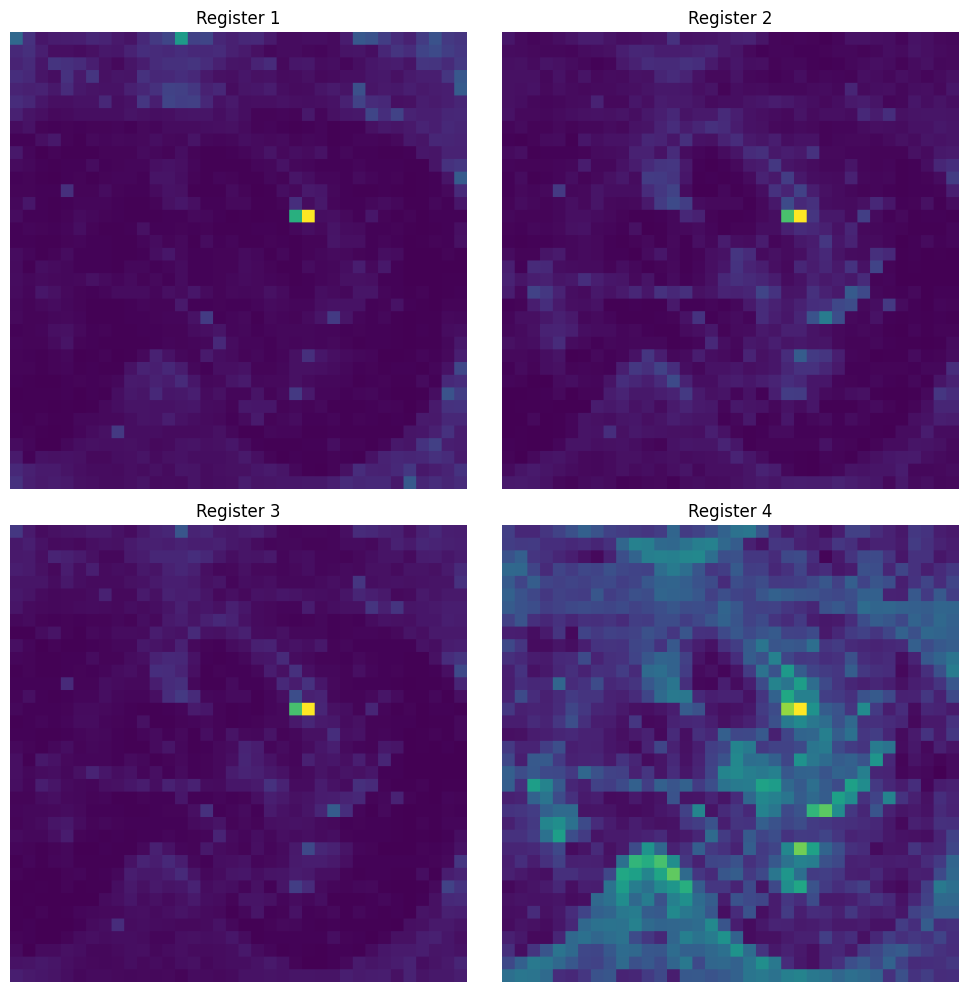
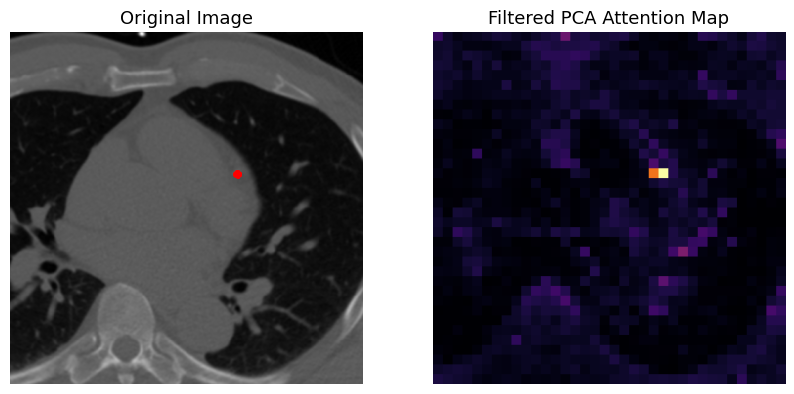